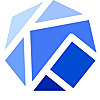
Kubeflow Blog
38 FOLLOWERS
This blog is hosted on GitHub Pages, via the kubeflow/blog repo. We are helping make ML on Kubernetes easy, portable and scalable, everywhere with our informative articles.
Kubeflow Blog
3M ago
We’re thrilled to announce the results of the 2023 Kubeflow Steering Committee (KSC) election.
The Kubeflow community has shown a strong commitment to the project’s future by casting their votes for the new leadership. Please welcome Yuan (Terry) Tang (Red Hat), Andrey Velichkevich (Apple), and Johnu George (Nutanix) who will be joining Josh Bottom (Consultant) and James Wu (Google) as KSC members. The three new members will serve a two-year term ending in 2025, beginning immediately.
The election saw a turnout of 72.88%, with 43 out of 59 eligible voters participating. The three nominees were ..read more
Kubeflow Blog
4M ago
The Kubeflow Community, known for its dedication to making machine learning workflows on Kubernetes simple, portable, and scalable, has recently announced a significant milestone in its journey. For the first time, they are holding elections for the Kubeflow Steering Committee (KSC)!
The KSC will be crucial in guiding the project’s direction, ensuring it continues to meet the needs of its growing user base. Candidates for this committee are drawn from the community’s diverse members, embodying the spirit of open-source collaboration that Kubeflow cherishes. This election marks a new chapter in ..read more
Kubeflow Blog
6M ago
Kubeflow v1.8’s powerful workflows uniquely deliver Kubernetes-native MLOps, which dramatically reduce yaml wrangling. ML pipelines are now constructed as modular components, enabling easily chainable and reusable ML workflows. The new Katib SDK reduces manual configuration and simplifies the delivery of your tuned model. v1.8 also introduces the PVC Viewer for easier persistent storage management eliminating the need for Kubernetes CLI storage commands.
Kubeflow 1.8 adds ARM processor support which simplifies adoption for Apple Silicon users and IoT servers. 1.8 delivers updated Tensorflow, P ..read more
Kubeflow Blog
9M ago
In April 2023, the Kubeflow user survey opened, gathering community feedback. The survey aimed to comprehend the adoption of Kubeflow and collect input on the benefits, gaps, and requirements for machine learning use cases.
The survey consisted 21 questions, including multiple-choice and freeform formats. It ran from April 11th to May 26th and received 90 responses. The 2023 survey yielded more targeted and actionable freeform answers, which provided further insights into users’ requirements and potential enhancements for Kubeflow. Furthermore, we received a significant amount of positive feed ..read more
Kubeflow Blog
1y ago
The Kubeflow Community is excited to announce the availability of the Kubeflow v1.6 software release, which includes support for Kubernetes v1.22 and introduces an alpha release of the Kubeflow Pipeline v2 (KFP v2) functionality.
Kubeflow v1.6 also adds new hyperparameter support for the population based algorithm in Katib, and provides a combined Python SDK for PyTorch, MXNet, MPI, XGBoost in Kubeflow’s distributed Training Operator. For model serving, v1.6 has new ClusterServingRuntime and ServingRuntime CRDs, and a new Model Spec was introduced to the InferenceService Predictor Spec, which ..read more
Kubeflow Blog
1y ago
In May 2022, the Kubeflow user survey was opened to gather community feedback. The goal of the survey was to understand the adoption of Kubeflow and to gather input on the benefits, gaps, and requirements for machine learning use cases.
The survey was comprised of 24 questions (multiple choice and freeform). It ran from May 6th to June 7th and received 151 responses. Out of the 151 responses, 91 responses provided optional feedback on how to improve Kubeflow and the Community. In the 2022 survey, we received an increased number of freeform answers, which provided additional insights into users ..read more
Kubeflow Blog
1y ago
The Kubeflow Community is excited to announce the release of Kubeflow 1.5.1. The Kubeflow 1.5.1 release provides a valuable enhancement to the caching feature of Kubeflow Pipeline (KFP) for any Kubernetes distribution with strict security policies for user application certificates. Caching is one of the crucial features of KFP which enables skipping a step that has already been executed which saves time and money. This release unblocks the caching feature to be used on distributions like AWS, the Mercedes Benz cluster, etc.
Kubeflow 1.5.1 includes the enhancements for the Kubeflow Pipelines ca ..read more
Kubeflow Blog
1y ago
The Kubeflow v1.5 software release improves ML model accuracy, lowers infrastructure costs, and simplifies operations by providing a more consistent user experience between components.
Lower infrastructure costs and improve model accuracy
Several enhancements in Kubeflow v1.5 lower infrastructure costs and help improve model accuracy. For example, new elastic training augments the Kubeflow training operator for PyTorch and enables PyTorch workers to be scaled up and down, providing fault tolerant and elastic training. This allows the training jobs to continue without restarting from scratch ev ..read more
Kubeflow Blog
1y ago
The Kubeflow Training Operator Working Group introduced several enhancements in the recent Kubeflow 1.4 release. The most significant was the introduction of the new unified training operator that enables Kubernetes custom resources (CR) for many of the popular training frameworks: Tensorflow, Pytorch, MXNet and XGboost. In addition, the tf-operator repository has been renamed to training-operator.
This single operator provides several valuable benefits:
Better resource utilisation - For releases prior to 1.4, each framework had a separate corresponding controller managing its distributed job ..read more
Kubeflow Blog
1y ago
The Kubeflow 1.4 release lays several important building blocks for the use of advanced metadata workflows. A quick summary of 1.4’s top deliveries includes:
Advanced metadata workflows with improved metric visualization and pipeline step caching in Kubeflow Pipelines (KFP) via the KFP Software Development Kit (SDK)
A new KFServing model user interface that displays ML model status, configuration, yaml, logs, and metrics
New Optuna Suggestion Service with multivariate TPE algorithm and Sobol’s Quasirandom Sequence support for hyperparameter tuning
A new, unified training operator that support ..read more