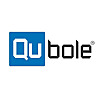
Qubole Blog » Data Engineering
2 FOLLOWERS
Cloud-native Big Data Activation Platform
Qubole Blog » Data Engineering
4M ago
Digital data is all around us. As per DataReportal, a total of 5.19 billion people around the world were using the internet at the start of Q3 2023, equivalent to 64.5 percent of the world’s total population. Internet users continue to grow too, with the latest data indicating that the world’s connected population grew by more than 100 million users in the 12 months to July 2023. If utilized correctly, data offers a vast number of opportunities to individuals and companies looking to improve their business intelligence, operational efficiency, profitability, and growth over time.
With this muc ..read more
Qubole Blog » Data Engineering
4M ago
When Hadoop is deployed with on-premises architecture, compute and storage are combined together. As a result, compute and storage must be scaled together and the clusters must be persistently on otherwise the data becomes inaccessible. On the cloud, compute and storage can be separated with a service such as EC2 and S3 used as the object-store. This means they can be scaled separately depending on the data team’s needs.
Why does this distinction matter? Since compute and storage are tied together in an on-premises solution, elasticity is much harder to achieve and manage. On the other hand ..read more
Qubole Blog » Data Engineering
4M ago
Today we reported some impressive stats on our growth in 2014. In short, last year was a phenomenal year for the company.
The amount of data our clients processed on Qubole in 2014 soared to 519 petabytes of data, compared to 34 petabytes in 2013. In fact, we’re now processing more than 100 petabytes per month. Customers logged more than 40 million compute hours on Qubole during the year, and the average monthly compute hours grew nearly 350% to 5.7 million per month.
These are extraordinary numbers that show
1.) How scalable our big data as a service platform is, and
2.) How valuable Qubole i ..read more
Qubole Blog » Data Engineering
4M ago
Poaching continues to be a significant problem throughout the world, specifically in Africa. Every year thousands of different animals are illegally hunted, many of which are endangered and on the brink of extinction. In an effort to fight this problem, scientists have gone to great lengths to better understand these creatures. They study them intensively in the hopes of better understanding their behavior and predicting their actions. With all this information, scientists can better understand where these animals travel, when they’ll be the most vulnerable, and take appropriate action to kee ..read more
Qubole Blog » Data Engineering
4M ago
Background
The Accenture Technology Labs Hadoop Deployment Comparison study recently stated something that we at Qubole have known for a long time, an investment in Hadoop-as-a-Service has many advantages over implementing a bare-metal Hadoop cluster.
The study used Accenture’s Data Platform Benchmark, to assess the Total Cost of Ownership for both solutions. This method of analysis has thrown up a more useful conclusion than if it has been looking at more limited metrics.
Approach
The approach was thorough and well-executed using a cluster with a client node, a primary NameNode, a secondary ..read more
Qubole Blog » Data Engineering
4M ago
Hive on Hadoop makes data processing so straightforward and scalable that we can easily forget to optimize our Hive queries. Well-designed tables and queries can greatly improve your query speed and reduce processing costs. This article includes five tips, which are valuable for ad-hoc queries, to save time, as much as for regular ETL (Extract, Transform, Load) workloads, to save money. The three areas in which we can optimize our Hive utilization are:
Data Layout (Partitions and Buckets)
Data Sampling (Bucket and Block sampling)
Data Processing (Bucket Map Join and Parallel execution)
We w ..read more
Qubole Blog » Data Engineering
1y ago
This post was originally published in July 2015 and has since been expanded and updated.
When it comes to big data tools, Apache Spark is quickly gaining steam both in the headlines and in real-world adoption. UC Berkeley’s AMPLab developed Spark in 2009 and open-sourced it in 2010. Since then, it has grown to become one of the largest open source communities in big data with over 200 contributors from more than 50 organizations. This open-source analytics engine stands out for its ability to process large volumes of data significantly faster than MapReduce because data is persisted in memory ..read more
Qubole Blog » Data Engineering
1y ago
By now, most businesses understand the appeal of using big data analytics. With big data, companies can improve their efficiency, increase productivity, and gain valuable insights that drive their work forward. Few will deny the important role big data now plays in organizations all over the world, but gaining those unique benefits requires having high-quality data, something that has become increasingly difficult to do. All too often, the data collected by businesses is filled with mistakes, errors, and incomplete values. This is referred to as dirty data, and it can represent a formidable o ..read more
Qubole Blog » Data Engineering
1y ago
A common characteristic of many analytics queries is that they are mostly invariant in form and function. Over multiple invocations of the query or command, one would find that only the range of inputs varies in the form of a couple of inputs, while the major part of the query remains the same.
Command templates in QDS have basically parameterized commands designed to effectively use this attribute to your advantage. Until now, to run the same command again with different inputs, you had to edit it, search for the fields in the (possibly huge) command and then modify them. With command templat ..read more
Qubole Blog » Data Engineering
1y ago
A 2018 Gartner article discussed the necessity of data lakes when it comes to implementing big data, stating “the fact remains that more than 80 percent of all data is unstructured. As more businesses turn to big data for future opportunities, the application of data lakes will rise.” This is a message that cloud companies like Amazon Web Services (AWS) embraced early on, focusing on infrastructure that could service architecture models with a large variety and volume of data as well as bursty and unpredictable compute needs.
This market trend has evidently caught on. Average IT salaries incre ..read more