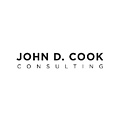
John D. Cook » Probability & Statistics
26 FOLLOWERS
Companies come to us for solutions to problems in applied math and data privacy. The need for these skills cuts across industries, and we have especially helped clients working in software, biotech, and law. Explore our articles on probability and statistics and how it can help you with your business.
John D. Cook » Probability & Statistics
1M ago
Surprise index
Warren Weaver [1] introduced what he called the surprise index to quantify how surprising an event is. At first it might seem that the probability of an event is enough for this purpose: the lower the probability of an event, the more surprise when it occurs. But Weaver’s notion is more subtle than this.
Let X be a discrete random variable taking non-negative integer values such that
Then the surprise index of the ith event is defined as
Note that if X takes on values 0, 1, 2, … N−1 all with equal probability 1/N, then Si = 1, independent of N. If N is very large, each outcome ..read more
John D. Cook » Probability & Statistics
1M ago
How would you estimate the size of an author’s vocabulary? Suppose you have a analyzed the author’s available works and found n words, x of which are unique. Then you know the author’s vocabulary was at least x, but it’s reasonable to assume that the author may have know words he never used in writing, or that at least not in works you have access to.
Brainerd [1] suggested the following estimator based on a Markov chain model of language. The estimated vocabulary is the number N satisfying the equation
The left side is a decreasing function of N, so you could solve the equation by findi ..read more
John D. Cook » Probability & Statistics
2M ago
There are many answers to the question in the title: How likely is a random variable to be far from its center?
The answers depend on how much you’re willing to assume about your random variable. The more you can assume, the stronger your conclusion. The answers also depend on what you mean by “center,” such as whether you have in mind the mean or the mode.
Chebyshev’s inequality says that the probability of a random variable X taking on a value more than k standard deviations from its mean is less than 1/k². This of course assumes that X has a mean and a standard deviation.
If we as ..read more
John D. Cook » Probability & Statistics
3M ago
I was thinking about the work I did when I worked in biostatistics at MD Anderson. This work was practical rather than mathematically elegant, useful in its time but not of long-term interest. However, one result came out of this work that I would call elegant, and that was a symmetry I found.
Let X be a beta(a, b) random variable and let Y be a beta(c, d) random variable. Let g(a, b, c, d) be the probability that a sample from X is larger than a sample from Y.
g(a, b, c, d) = Prob(X > Y)
This function often appeared in the inner loop of a simulation and so we spent thousands of CPU-hours c ..read more
John D. Cook » Probability & Statistics
6M ago
Differential privacy can be rigid and overly conservative in practice, and so finding ways to relax pure differential privacy while retaining its benefits is an active area of research. Two approaches to doing this are concentrated differential privacy [1] and Rényi differential privacy [3].
Differential privacy quantifies the potential impact of an individual’s participation or lack of participation in a database and seeks to bound the difference. The original proposal for differential privacy and the approaches discussed here differ in how they measure the difference an individual can make ..read more
John D. Cook » Probability & Statistics
6M ago
Probability density functions are independent of physical units. The normal distribution, for example, works just as well when describing weights or times. But sticking in units anyway is useful.
Normal distribution example
Suppose you’re trying to remember the probability density function for the normal distribution. Is the correct form
or
or
or maybe some other variation?
Suppose the distribution represents heights. (More on that here, here, and here.) The argument to an exponential function must be dimensionless, so the numerator and denominator in the exp() argument must have the same u ..read more
John D. Cook » Probability & Statistics
6M ago
Differential privacy protects user privacy by adding randomness as necessary to the results of queries to a database containing private data. Local differential privacy protects user privacy by adding randomness before the data is inserted to the database.
Using the visualization from this post, differential privacy takes the left and bottom (blue) path through the diagram below, whereas local differential privacy takes the top and right (green) path.
The diagram does not commute. Results are more accurate along the blue path. But this requires a trusted party to hold the identifiable data. L ..read more
John D. Cook » Probability & Statistics
6M ago
There are many ways to describe the distance between two probability distributions. The previous two posts looked at using the p-norm to measure the difference between the PDFs and using Kullbach-Leibler divergence. Earth mover’s distance (EMD) is yet another approach.
Imagine a probability distribution on ℝ² as a pile of dirt. Earth mover’s distance measures how different two distributions are by how much work it would take to reshape the pile of dirt representing one distribution into a pile of dirt representing the other distribution. Unlike KL divergence, earth mover’s distance is symmetri ..read more
John D. Cook » Probability & Statistics
6M ago
The previous post looked at the best approximation to a normal density by normal density with a different mean. Dan Piponi suggested in the comments that it would be good to look at the Kullback-Leibler (KL) divergence.
The previous post looked at the difference from between two densities from an analytic perspective, solving the problem that an analyst would find natural. This post takes an information theoretic perspective. Just is p-norms are natural in analysis, KL divergence is natural in information theory.
The Kullback-Leibler divergence between two random variables X and Y is ..read more
John D. Cook » Probability & Statistics
6M ago
In my previous post on approximating a logistic distribution with a normal distribution I accidentally said something about approximating a normal with a normal.
Obviously the best approximation to a probability distribution is itself. As Norbert Wiener said “The best material model of a cat is another, or preferably the same, cat.”
But this made me think of the following problem. Let f be the density function of a standard normal random variable, i.e. one with mean zero and standard deviation 1. Let g be the density function of a normal random variable with mean μ > 0 and standard deviatio ..read more