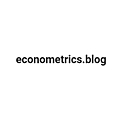
econometrics.blog
54 FOLLOWERS
This is a blog about econometrics, statistics, and related topics. I am Francis J. DiTraglia, an associate professor of Economics and a researcher. On this website, I will provide the information and insights related to my ongoing research model selection and averaging measurement error and models with social interactions.
econometrics.blog
10M ago
Here’s a puzzle for you. What will happen if we regress some outcome of interest on both an endogenous regressor and a valid instrument for that regressor? I hadn’t thought about this question until 2018, when one of my undergraduate students asked it during class. If memory serves, my off-the-cuff answer left much to be desired.1 Five years later I’m finally ready to give a fully satisfactory answer; better late than never I suppose!
The Model
We’ll start by being a bit more precise about the setup. Suppose that \(Y\) is related to \(X\) according to the following linear causal model \[ Y \le ..read more
econometrics.blog
1y ago
To do well in an econometrics or statistics course at any level, you need to have a large number of simple properties of random variables at your fingertips. Some years back I made a handout containing the most important properties for my undergraduate students at the University of Pennsylvania. In the hopes that this might be of use to others, I’ve released an updated pdf on github. You can fork the repository here. If you spot any errors or want to suggest any additions, feel free to raise an issue or send me a pull request ..read more
econometrics.blog
1y ago
The Poisson distribution is the most famous probability model for counts, non-negative integer values. Many real-world phenomena are well approximated by this distribution, including the number of German bombs that landed in 1/4km grid squares in south London during WWII. Formally, we say that a discrete random variable \(X\) follows a Poisson distribution with rate parameter \(\mu > 0\), abbreviated \(X \sim \text{Poisson}(\mu)\), if \(X\) has support set \(\{0, 1, 2, ...\}\) and probability mass function \[ p(x) \equiv \mathbb{P}(X=x) = \frac{e^{-\mu }\mu^x}{x!}. \] Using some clever alge ..read more
econometrics.blog
1y ago
If you study enough econometrics, you will eventually come across an asymptotic argument in which some parameter is assumed to change with sample size. This peculiar notion goes by a variety of names including “Pitman drift,” a “sequence of local alternatives,” and “local mis-specification,” and crops up in a wide range of problems from weak instruments, to model selection, to power analysis.1 Whatever you choose to call it, the idea of a parameter that changes with sample size is bizarre, and I remember spending weeks trying to understand it when I was a graduate student. How could parameters ..read more
econometrics.blog
1y ago
In this post we’ll examine a very simple instrumental variables model from three different perspectives: two familiar and one a bit more exotic. While all three yield the same solution in this particular model, they lead in different directions in more complicated examples. Crucially, each gives us a different way of thinking about the problem of endogeneity and how to solve it.
The Setup
Consider a simple linear causal model of the form \(Y \leftarrow \alpha + \beta X + U\) where \(X\) is endogenous, i.e. related to the unobserved random variable \(U\). Our goal is to learn \(\beta\), th ..read more
econometrics.blog
1y ago
“Suppose that \(Y = \alpha + \beta X + U\).” A sentence like this is bound to come up dozens of times in an introductory econometrics course, but if I had my way it would be stamped out completely. Without further clarification, this sentence could mean any number of different things. Even with clarification, it is a source of endless confusion for beginning students. What is \(U\) exactly? What is the meaning of “\(=\)” in this context? We can do better. Here are a few suggestions.
Population Linear Regression
Sometimes \(Y = \alpha + \beta X + U\) is nothing more than the population linear r ..read more
econometrics.blog
1y ago
Pop Quiz: If \(D^*\) and \(D\) are binary random variables and \(D\) is a noisy measure of \(D^*\), is it possible for the measurement error \(W \equiv D - D^*\) to be classical? Explain why or why not. (Answer below)
Classical Measurement Error
Classical measurement error is a problem that is easy to understand and relatively easy to address. Roughly speaking, classical measurement error refers to a situation in which the variable we observe equals the truth plus noise \[ \text{Observed} = \text{Truth} + \text{Noise} \] where the noise is unrelated to the truth and “everything else.” (I’ll be ..read more
econometrics.blog
1y ago
The F-statistic for a test of multiple linear restrictions is a staple of introductory econometrics courses. In the simplest case, it can be written as \[F \equiv \frac{(SSR_r - SSR_{u})/q}{SSR_{u} / (n - k - 1)}\] where \(SSR_r\) is the restricted sum of squared residuals, \(SSR_{u}\) is the unrestricted sum of squared residuals, \(q\) is the number of restrictions, and \((n - k - 1)\) is the degrees of freedom of the unrestricted model.1
In my experience, students encountering this expression for the first time find it bewilderingly arbitrary; it becomes just one more item to add the a list ..read more
econometrics.blog
1y ago
On a recent exam I asked students to extend the logic of propensity score weighting to handle a treatment that takes on three rather than two values: basically a stripped-down version of Imbens (2000). Nearly everyone figured this out without much trouble, which is good news! At the same time, I noticed some common misconceptions about the all-important selection-on-observables assumption: \[ \mathbb{E}[Y_0|D,X] = \mathbb{E}[Y_0|X] \quad \text{and} \quad \mathbb{E}[Y_1|D,X] = \mathbb{E}[Y_1|X] \] where \((Y_0, Y_1)\) are the potential outcomes corresponding to a binary treatment \(D\) and \(X ..read more
econometrics.blog
1y ago
The following is a piece I wrote for the LMH News, based on a general interest webinar that I gave in November of 2020. If this post inspires you to learn more about causal inference, you may enjoy browsing my teaching materials on treatment effects.
Will earning a PPE degree from Oxford increase your lifetime earnings? Does eating bacon sandwiches cause cancer? Does watching Fox News make you vote Republican? Will owning a dog increase your lifespan? Each of these questions concerns the causal effect of a treatment on an outcome. In social science, a “treatment” is any factor whose causal eff ..read more