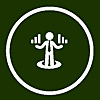
Eran Raviv Blog » Statistics and Econometrics
54 FOLLOWERS
check my blogs on the topic of Modern statistics and econometrics with applications to financial markets. I am Eran Raviv, working for a large pension fund manager and the author of this blog. Here, you can read about topics I think I want to know more about, or topics I feel there is something to be said about, not easily found elsewhere.
Eran Raviv Blog » Statistics and Econometrics
1M ago
Remarkably, considering that correlation modelling dates back to 1890, statisticians still make meaningful progress in this area. A recent step forward is given in A New Coefficient of Correlation by Sourav Chatterjee. I wrote about it shortly after it came out, and it has since garnered additional attention and follow-up results. The more I read about it, the more I am impressed with it. This post provides some additional details based on recent research.
What is Chatterjee’s rank correlation?
The formula for Chatterjee’s rank correlation:
is the rank of and is rearranged to ..read more
Eran Raviv Blog » Statistics and Econometrics
3M ago
AI algorithms are in the air. The success of those algorithms is largely attributed to dimension expansions, which makes it important for us to consider that aspect.
Matrix multiplication can be beneficially perceived as a way to expand the dimension. We begin with a brief discussion on PCA. Since PCA is predominantly used for reducing dimensions, and since you are familiar with PCA already, it serves as a good springboard by way of a contrasting example for dimension expansion. Afterwards we show some basic algebra via code, and conclude with a citation that provides the intuition for the rea ..read more
Eran Raviv Blog » Statistics and Econometrics
4M ago
Welcome 2024.
This blog is just a personal hobby. When I’m extra busy as I was this year the blog is a front-line casualty. This is why 2023 saw a weaker posting stream. Nonetheless I am pleased with just over 30K visits this year, with an average of roughly one minute per visit (engagement time, whatever google-analytics means by that). This year I only provide the top two posts (rather than the usual 3). Both posts have to do with statistical shrinkage:
The one is Statistical Shrinkage (2) and the other is Statistical Shrinkage (3).
On the left (scroll down) you can find the most popular po ..read more
Eran Raviv Blog » Statistics and Econometrics
4M ago
Matrix multiplication is a fundamental computation in modern statistics. It’s at the heart of all concurrent serious AI applications. The size of the matrices nowadays is gigantic. On a good system it takes around 30 seconds to estimate the covariance of a data matrix with dimensions , a small data today’s standards mind you. Need to do it 10000 times? wait for roughly 80 hours. Have larger data? running time grows exponentially. Want a more complex operation than covariance estimate? forget it, or get ready to dig deep into your pockets.
We, mere minions who are unable to splurge thousands of ..read more
Eran Raviv Blog » Statistics and Econometrics
5M ago
A common issue encountered in modern statistics involves the inversion of a matrix. For example, when your data is sick with multicollinearity your estimates for the regression coefficient can bounce all over the place.
In finance we use the covariance matrix as an input for portfolio construction. Analogous to the fact that variance must be positive, covariance matrix must be positive definite to be meaningful. The focus of this post is on understanding the underlying issues with an unstable covariance matrix, identifying a practical solution for such an instability, and connecting that solut ..read more
Eran Raviv Blog » Statistics and Econometrics
5M ago
Imagine you’re picking from 1,000 money managers. If you test just one, there’s a 5% chance you might wrongly think they’re great. But test 10, and your error chance jumps to 40%. To keep your error rate at 5%, you need to control the “family-wise error rate.” One method is to set higher standards for judging a manager’s talent, using a tougher t-statistic cut-off. Instead of the usual 5% cut (t-stat=1.65), you’d use a 0.5% cut (t-stat=2.58).
When testing 1,000 managers or strategies, the challenge increases. You’d need a manager with an extremely high t-stat of about 4 to stay within the 5% e ..read more
Eran Raviv Blog » Statistics and Econometrics
9M ago
During 2017 I blogged about Statistical Shrinkage. At the end of that post I mentioned the important role signal-to-noise ratio (SNR) plays when it comes to the need for shrinkage. This post shares some recent related empirical results published in the Journal of Machine Learning Research from the paper Randomization as Regularization. While mainly for tree-based algorithms, the intuition undoubtedly extends to other numerical recipes also.
While bootstrap aggregation (bagging) use all explanatory variables in the creation of the forecast, the random forest (RF from hereon) algorithms choose o ..read more
Eran Raviv Blog » Statistics and Econometrics
1y ago
Tree-based methods like decision trees and their powerful random forest extensions are one of the most widely used machine learning algorithms. They are easy to use and provide good forecasting performance off the cuff more or less. Another machine learning community darling is the deep learning method, particularly neural networks. These are ultra flexible algorithms with impressive forecasting performance even (and especially) in highly complex real-life environments.
This post is shares:
Two academic references lauding the powerful performance of tree-based methods.
Because both neural net ..read more
Eran Raviv Blog » Statistics and Econometrics
1y ago
The word spurious refers to “outwardly similar or corresponding to something without having its genuine qualities.” Fake.
While the meanings of spurious correlation and spurious regression are common knowledge nowadays, much less is understood about spurious factors. This post draws your attention to recent, top-shelf, research flagging the risks around spurious factor analysis. While formal solutions are still pending there are couple of heuristics we can use to detect possible problems.
Since you know what spurious correlation is, it’s easy to board the train of thought at this station. When ..read more
Eran Raviv Blog » Statistics and Econometrics
1y ago
Hyper-parameters are parameters which are not estimated as an integral part of the model. We decide on those parameters but we don’t estimate them within, but rather beforehand. Therefore they are called hyper-parameters, as in “above” sense.
Almost all machine learning algorithms have some hyper-parameters. Data-driven choice of hyper-parameters means typically, that you re-estimate the model and check performance for different hyper-parameters’ configurations. This adds considerable computational burden. One popular approach to set hyper-parameters is based on a grid-search over possible val ..read more