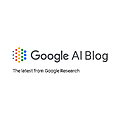
Google AI Blog
7,026 FOLLOWERS
Google AI is focused on bringing the benefits of AI to everyone. In conducting and applying our research, we advance the state-of-the-art in many domains.
The latest news and updates directly from Google bring the benefits of AI to everyone.
Google AI Blog
4M ago
Posted by Lizao (Larry) Li, Software Engineer, and Rob Carver, Research Scientist, Google Research
Accurate weather forecasts can have a direct impact on people’s lives, from helping make routine decisions, like what to pack for a day’s activities, to informing urgent actions, for example, protecting people in the face of hazardous weather conditions. The importance of accurate and timely weather forecasts will only increase as the climate changes. Recognizing this, we at Google have been investing in weather and climate research to help ensure that the forecasting technology of tomorrow can ..read more
Google AI Blog
4M ago
Posted by Urs Köster, Software Engineer, Google Research
Time series problems are ubiquitous, from forecasting weather and traffic patterns to understanding economic trends. Bayesian approaches start with an assumption about the data's patterns (prior probability), collecting evidence (e.g., new time series data), and continuously updating that assumption to form a posterior probability distribution. Traditional Bayesian approaches like Gaussian processes (GPs) and Structural Time Series are extensively used for modeling time series data, e.g., the commonly used Mauna Loa CO2 dataset. However ..read more
Google AI Blog
4M ago
Posted by Atilla Kiraly, Software Engineer, and Rory Pilgrim, Product Manager, Google Research
Lung cancer is the leading cause of cancer-related deaths globally with 1.8 million deaths reported in 2020. Late diagnosis dramatically reduces the chances of survival. Lung cancer screening via computed tomography (CT), which provides a detailed 3D image of the lungs, has been shown to reduce mortality in high-risk populations by at least 20% by detecting potential signs of cancers earlier. In the US, screening involves annual scans, with some countries or cases recommending more or less frequent ..read more
Google AI Blog
4M ago
Posted by Yossi Matias, VP Engineering & Research, and Grey Nearing, Research Scientist, Google Research
Floods are the most common natural disaster, and are responsible for roughly $50 billion in annual financial damages worldwide. The rate of flood-related disasters has more than doubled since the year 2000 partly due to climate change. Nearly 1.5 billion people, making up 19% of the world’s population, are exposed to substantial risks from severe flood events. Upgrading early warning systems to make accurate and timely information accessible to these populations can save thousands of l ..read more
Google AI Blog
4M ago
Posted by Srinivas Sunkara and Gilles Baechler, Software Engineers, Google Research
Screen user interfaces (UIs) and infographics, such as charts, diagrams and tables, play important roles in human communication and human-machine interaction as they facilitate rich and interactive user experiences. UIs and infographics share similar design principles and visual language (e.g., icons and layouts), that offer an opportunity to build a single model that can understand, reason, and interact with these interfaces. However, because of their complexity and varied presentation formats, infographics a ..read more
Google AI Blog
4M ago
Posted by Pooja Rao, Research Scientist, Google Research
Health datasets play a crucial role in research and medical education, but it can be challenging to create a dataset that represents the real world. For example, dermatology conditions are diverse in their appearance and severity and manifest differently across skin tones. Yet, existing dermatology image datasets often lack representation of everyday conditions (like rashes, allergies and infections) and skew towards lighter skin tones. Furthermore, race and ethnicity information is frequently missing, hindering our ability to assess di ..read more
Google AI Blog
4M ago
Posted by Mike Schaekermann, Research Scientist, Google Research, and Ivor Horn, Chief Health Equity Officer & Director, Google Core
Health equity is a major societal concern worldwide with disparities having many causes. These sources include limitations in access to healthcare, differences in clinical treatment, and even fundamental differences in the diagnostic technology. In dermatology for example, skin cancer outcomes are worse for populations such as minorities, those with lower socioeconomic status, or individuals with limited healthcare access. While there is great promise in rec ..read more
Google AI Blog
4M ago
Posted by Yun Zhu and Lijuan Liu, Software Engineers, Google Research
Large language model (LLM) advancements have led to a new paradigm that unifies various natural language processing (NLP) tasks within an instruction-following framework. This paradigm is exemplified by recent multi-task LLMs, such as T0, FLAN, and OPT-IML. First, multi-task data is gathered with each task following a task-specific template, where each labeled example is converted into an instruction (e.g., "Put the concepts together to form a sentence: ski, mountain, skier”) paired with a corresponding response (e.g., "Ski ..read more
Google AI Blog
4M ago
Posted by Bahare Fatemi and Bryan Perozzi, Research Scientists, Google Research
Imagine all the things around you — your friends, tools in your kitchen, or even the parts of your bike. They are all connected in different ways. In computer science, the term graph is used to describe connections between objects. Graphs consist of nodes (the objects themselves) and edges (connections between two nodes, indicating a relationship between them). Graphs are everywhere now. The internet itself is a giant graph of websites linked together. Even the knowledge search engines use is organized in a graph ..read more
Google AI Blog
5M ago
Posted by Dave Steiner, Clinical Research Scientist, Google Health, and Rory Pilgrim, Product Manager Google Research
There’s a worldwide shortage of access to medical imaging expert interpretation across specialties including radiology, dermatology and pathology. Machine learning (ML) technology can help ease this burden by powering tools that enable doctors to interpret these images more accurately and efficiently. However, the development and implementation of such ML tools are often limited by the availability of high-quality data, ML expertise, and computational resources.
One way to ca ..read more