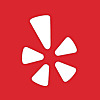
Yelp Engineering Blog
1,596 FOLLOWERS
Read news and blog posts to see the technical side of Yelp Engineering. Our engineers have shared their thoughts with the world on our blog. Take a peek into our engineering & product teams and all the work that we do. Yelp connects people with great local businesses.
Yelp Engineering Blog
1M ago
One of Yelp’s top priorities is the trust and safety of our users. Yelp’s platform is most well-known for its reviews, and its moderation practices have been recognised in academic research for mitigating misinformation and building consumer trust. In addition to reviews, Yelp’s Trust and Safety team takes significant measures when it comes to protecting its users from inappropriate material posted through other content types. This blog post discusses how Yelp protects its users from inappropriate content in videos. Videos at Yelp Recently, Yelp revamped its review experience by giving users t ..read more
Yelp Engineering Blog
1M ago
Yelp develops two major applications, Yelp & Yelp for Business, for Web (Desktop & Mobile), iOS, and Android platforms. That’s eight unique clients! Keeping a fresh, consistent UI on all these clients is a major challenge. Server-driven UI (SDUI) has become a standard industry technique for managing UI on multiple platforms. At Yelp, many product teams created SDUI frameworks for their features. Though successful, these frameworks were expensive to develop and maintain, and no single SDUI framework supported all our clients. In late 2021, we began building a unified SDUI framework call ..read more
Yelp Engineering Blog
1M ago
What is Engineering Effectiveness Metrics (EE Metrics)? EE Metrics was envisioned as a hub that helps teams manage their technical debt. EE Metrics provides every team with a detailed web page that contains information about technical debt that needs to be addressed. It also serves as a platform to highlight top engineering initiatives at the organization level. EE Metrics empowers infrastructure teams to surface important migrations or metrics that could improve the health of software projects. Organization-wide migrations of technologies can often be difficult to surface and keep track of. G ..read more
Yelp Engineering Blog
1M ago
Yelp’s mission is to connect consumers with great local businesses by giving them access to reliable and useful information. Consumer trust is one of our top priorities, which is why we make significant investments in technology and human moderation to protect the integrity and quality of content on Yelp. As a platform for user-generated content, we rely on our community of users and business owners to help report reviews that they believe may violate our Terms of Service and Content Guidelines. Our User Operations team investigates all flagged content and, if it’s found to be in violation of ..read more
Yelp Engineering Blog
1M ago
Yelp relies heavily on streaming to synchronize enormous volumes of data in real time. This is facilitated by Yelp’s underlying data pipeline infrastructure, which manages the real-time flow of millions of messages originating from a plethora of services. This blog post covers how we leverage Yelp’s extensive streaming infrastructure to build robust data abstractions for our offline and streaming data consumers. We will use Yelp’s Business Properties ecosystem (explained in the upcoming sections) as an example. Key terminology Let’s start by covering certain key terms used throughout the post ..read more
Yelp Engineering Blog
7M ago
While we once used Elasticsearch at Yelp, we have since built a replacement called Nrtsearch. The benefits and motivations of this switch can be found in our blog post: Nrtsearch: Yelp’s Fast, Scalable and Cost Effective Search Engine. However in this blog post, we will discuss the motivations behind building Nrtsearch Coordinator - a gateway for Nrtsearch clusters. We will also go over how Nrtsearch Coordinator adds sharding logic to Nrtsearch, handles scatter-gather queries, and adds support for dark/live launching cluster improvements. Motivations We traditionally used a gateway to call Ela ..read more
Yelp Engineering Blog
9M ago
At Yelp, Apache Spark and JupyterHub are heavily used for batch processing and interactive use-cases, such as in building feature models, conducting ad-hoc data analysis, sharing templates, making on-boarding materials, creating visualizations, and producing sales reports. Our initial deployments of Jupyter at Yelp were iPython notebooks managed at an individual level. Later on when Jupyterlab was released (2018), our notebook ecosystem was extended to Jupyter Servers running on dev boxes, which was managed by individual engineering teams. Over time with growing use-cases and data-flow, this i ..read more
Yelp Engineering Blog
10M ago
Merging code safely can be quite time consuming for busy repositories. A common method is to test and merge branches serially, and one at a time, in order to ensure the safety of the main branch. However, this method does not scale well when many developers want to merge code at the same time. In this blog post, you’ll see how we’ve sped up code merging at Yelp by creating a batched merge queue system! Why Merge Queues? In our blog post about Gondola, our frontend Platform as a Service (PaaS), we talked about the benefits of moving to a ..read more
Yelp Engineering Blog
1y ago
Keeping project dependencies up to date is an ever-growing concern. An increasing number of dependencies is used for even the most simple applications. It’s easy for teams to deprioritize maintaining them, resulting in numerous security vulnerabilities. As dependencies become increasingly out of date, the level of effort to get a project into a good state increases significantly. Teams may even get blocked by outdated dependencies when doing critical development work. Being proactive about applying upgrades goes a long way. Tools like Dependabot can really help with this. But what if you’re tr ..read more
Yelp Engineering Blog
1y ago
In 2018, Yelp switched from using the MVP architecture to the MVI architecture for Android development. Since then, adoption of our new MVI architecture library has risen and we’ve seen some great performance and scalability wins. In this blog post, we’ll cover why we switched to MVI in the first place, how we managed to get performant screens by default, and our take on unit testing MVI. What is MVI? One of the main reasons to use an architecture is to make things easier to test by separating concerns. For Android, this means keeping the Android SDK out of our ..read more